In an era where social media content zips across the internet at the speed of light, ideas, words and phrases are generated at a staggering pace, leaving the uninitiated confounded. Therefore, I am not sure if many of us have heard the new additions to the English lexicon – deepfakes and shallowfakes, let alone understand what they mean.
Most of us are familiar with the deliberate spread of misinformation or partisan content, on social media, coined ‘fake news’, which is influencing political and social landscapes across the globe. The fake news devoid of realistic images or videos does not create the intended impact, as the old English adage says “a picture is worth a thousand words”. Until recently, a professional video editor with his team along with sophisticated video editing tools was essential to alter or recreate images. Techniques such as morphing using Photoshop is passé as it can easily discernible to even casual scrutiny. This limited the spread of authentic-looking fake images. However, in the last two years, technological advances and the ubiquitous internet have enabled the average John Doe to swiftly create and disseminate high quality tampered video, aided by an ever-growing social media.
“Shallowfakes” have been around for a while and refer to the numerous(tens of thousands of videos) circulated with malicious intent worldwide, which are not crafted with sophisticated AI, but often simply recycled, staged or re-edited and relabelled content uploaded on the net. It may be as simple as mislabelling content to discredit someone or spread false information. Shallowfakes have been in use in recent times with devastating effect as shown by the recent example of the doctored video of US House Speaker Nancy Pelosi that went viral and was retweeted by President Donald Trump.
It was only in 2017 that the world woke up to “deepfakes” when it was used as a moniker by a Reddit[1] user. Deepfake is a portmanteau of “deep learning” and “fake”. It started with an anonymous Reddit user posting digitally altered pornographic videos using machine-learning technology. While the user was banned by Reddit, it spurred a wave of copycats on other platforms. Experts believe there are now about 10,000 deepfake videos circulating online, and the number is growing.
The use of this machine learning technique hitherto was mostly limited to the AI research community in universities and it was only when the Reddit user started using Generative Adversarial Networks (GANs), that the common man became aware of its application to video and images. He was building GANs using TensorFlow, Google’s free open-source machine learning software, to superimpose celebrities’ faces on the bodies of women in pornographic movies.
However, for the people who think this is Geek (essentially anybody without a PhD in AI), it is a machine learning technique, invented by a graduate student, Ian Goodfellow, in 2014 as a method to algorithmically generate new types of data out of existing data sets. GAN is a class of machine learning systems where two neural networks contesting with each other in a game (in the sense of game theory, often but not always in the form of a zero-sum game). Given a training set, this technique learns to generate new data with the same statistics as the training set. Photo images are readily available online. In 2017, it was estimated that 1,000 selfies are uploaded to Instagram every second. The more pictures of the target, the more realistic the deep fake. GANs can also be used to generate new audio from existing audio, or new text from an existing text – it is a multi-use technology.
In plain English, in deepfake technology, GANs are trained to replicate patterns, such as the face of a person, which gradually improves the realism of the synthetically generated faces. Basically, it works like a cat-and-mouse game between two neural networks. One network, called “the generator,” is producing the fake video based on training data (real images), and the other network, “the discriminator,” is trying to distinguish between the real images and the fake video. This iterative process continues until the generator is able to fool the discriminator into thinking that the footage is real.
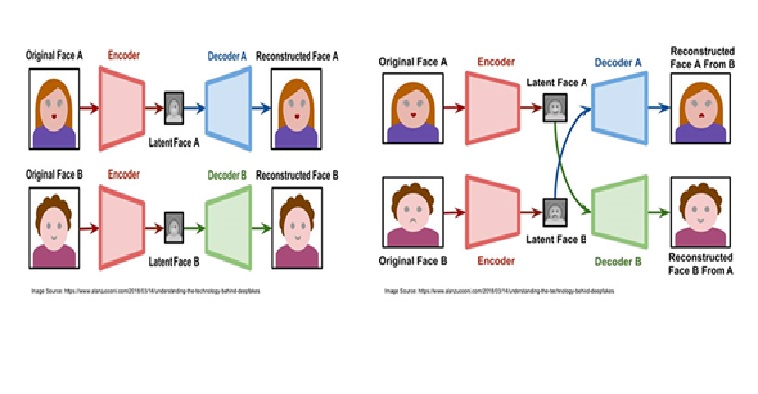
This was logically taken to the next level by the creator of the videos who released FakeApp, an easy-to-use platform for making forged media. The free software effectively democratized the power of GANs. Now, anyone with access to the internet and pictures of a person’s face could generate their own deep fake. The quality of the videos produced by the software beats the current state of the art face recognition systems based on VGG and Facenet neural networks, with 85.62% and 95.00% false acceptance rates (on high-quality versions) respectively[2].
On May 19, researchers at the Samsung AI Center in Moscow developed a way to create “living portraits” from a very small dataset—as few as one photograph, in some of their models. This, in a way, is a leap beyond what even deepfakes and other algorithms using generative adversarial networks can accomplish. Instead of teaching the algorithm to paste one face onto another using a catalogue of expressions from one person, they use the facial features that are common across most humans to then puppeteer a new face. The details of the process are based on the paper, “Few-Shot Adversarial Learning of Realistic Neural Talking Head Models,”. [3]
While altering pornographic content does not affect the public at large, however, in the future, deep fakes could be exploited by purveyors of “fake news” to create digital wildfires. There is always the danger of our networked information environment interacting in toxic ways with our cognitive biases based on fake news. Deep fakes will exacerbate this problem significantly. Anyone with access to this technology – from state-sanctioned propagandists to trolls – would be able to skew information, manipulate beliefs, and in so doing, push ideologically opposed online communities deeper into their own subjective realities.
To counter the growing stream of fake news, misinformation and deepfakes are tough as it is hard to keep up with. Relying on forensic detection alone to combat deep fakes is becoming less viable, due to the rate at which machine learning techniques can circumvent them. Work is in progress to develop forensic technology to identify digital forgeries, with new detection methods to counteract the spread of deep fakes. Techniques to identify subtle changes of colour that occur in the face as blood is pumped in and out are being explored, as the signal is so minute that the machine learning software is unable to pick it up – at least for now.
So there is hope that in the future, that deepfakes could be countered using the same AI, which helped to created it.
Title image courtesy: https://www.analyticsinsight.net/
References:
[1] Reddit is an American social news aggregation, web content rating, and discussion website, where registered members submit content to the site such as links, text posts, and images, which are then voted up or down by other members. Posts are organized by subject into user-created boards called “subreddits”, which cover a variety of topics including news, science, movies, video games, music, books, fitness, food, and image-sharing. Submissions with more up-votes appear towards the top of their subreddit and, if they receive enough votes, ultimately on the site’s front page. As of March 2019, Reddit had 542 million monthly visitors (234 million unique users), ranking as the No. 6 most visited website in U.S. and No. 21 in the world
[2] Pavel Korshunov and Sebastien Marcel; DeepFakes: a New Threat to Face Recognition? Assessment and Detection
[3]https://arxiv.org/abs/1905.08233, Egor Zakharov, Aliaksandra Shysheya, Egor Burkov, Victor Lempitsky, Few-Shot Adversarial Learning of Realistic Neural Talking Head Models
Disclaimer: The views and opinions expressed by the author do not necessarily reflect the views of the Government of India and Defence Research and Studies