The failure of Israeli intelligence to sense the mobilisation of Hamas attack on 07 Oct 2023 is a pity. Investigations are on by the Israel defence force (IDF) to identify the reasons for the failure of their Artificial Intelligence (AI) assisted Air Defence system, ‘Iron Dome’. Surrendering a nation’s security to AI has been questioned by many military commanders. Nations having advanced autonomous air-defence systems are rewriting their algorithms now to plug the existing loopholes.
Each of the short-range rockets fired by Hamas on 07 Oct 2023 costs between US$300 and US$800 in the market. The intercepting missiles of the Israel Defence Force (IDF) cost about US$40,000 to US$50,000 per piece. Is it worth maintaining such an expensive air defence system? Can we make it more fail safe?
The primary reason for the failure of Iron Dome could be its inability to trace and designate 5000+ rockets being launched by Hamas from multiple locations simultaneously within a short span of 30 minutes. Rockets fired in rapid succession might have become too much for the ‘Dome’ to handle. Another reason could be the introduction of new type of rockets by the Hamas for the attack, whose signature might not have been available in the library of targets of the Iron Dome system. Besides, Hamas mixed rockets with few loitering munitions during attack.
India has to consider many factors while deploying her indigenous air defence system. Currently, we are integrating hetro systems with multiple shields acquired from the east and west. AI plays a crucial role in enhancing the air defence capabilities. The development of a native algorithm post-integration is critical for the design and development of an indigenous system. Using non-kinetic methodologies instead of kinetic ones while countering the penetrating threats needs to be explored for better reliability. Let us explore how AI can aid each functionality of the air defence system.
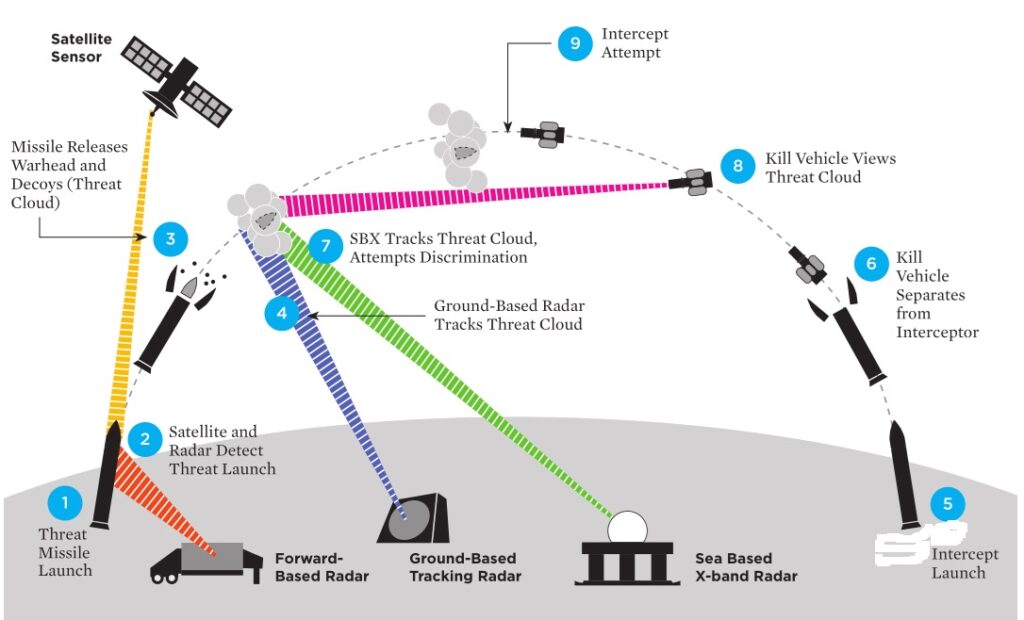
Automated Multi-Target Identification
AI has become common in radar systems to enhance capabilities, improve efficiency and enable more sophisticated functionalities. AI is used to process and analyse data from radar systems, improving the detection and tracking of aircraft and missiles. Air-defence systems have long-range to short-range radars other than the weapon radars used for counterattacks. Electronic Support Measure (ESM) systems have key sensors covering the entire spectrum of electromagnetic waves. These sensors are placed either on surveillance satellites/UAVs/aircraft or even on mountaintops or onboard warships. Both sensor and data fusions are incorporated to ascertain the existence of potential threats by analysing the output from surveillance radars and ESM sensors.
Algorithms can enhance multi-target identification simultaneously in a cluttered scenario. Machine learning techniques could enable radar systems to recognize complex patterns associated with different types of targets, improving the accuracy of target detection and classification. During the subsequent signal processing, AI algorithms can filter out noise and interference in the radar/ESM data, improving the signal-to-noise ratio and enhancing the system’s ability to detect targets in challenging environments. AI can even optimize beam-forming in radar systems by dynamically adjusting the directionality of the radar beams to focus on specific areas of interest. AI assistance can be sort while performing advanced signal processing in Synthetic and Inverse Synthetic Aperture Radars.
Labelling Airborne Targets
AI enables real-time processing of sensor data, allowing quick classification of airborne targets post-detection. Machine learning algorithms can help distinguish between friendly and hostile aircraft/ missiles, reduce false alarms and adapt to changing threat scenarios. Labelling of targets can be done during this phase. This may be done post comparison with the existing target /emitter library data. Training of systems need to be done with the historical / synthetic data to achieve a foolproof timely classification. If new targets are identified during this phase, a respective signature library is created as a part of learning for future comparison.
AI algorithms can analyze radar, sensor or imaging data to extract relevant features from airborne targets. These features may include shape, size, speed and other parameters that aid classification. Machine learning models excel at pattern recognition. They can be trained on vast datasets to recognize patterns in sensor data associated with different types of airborne targets, enabling accurate and rapid classification. Deep learning techniques, such as convolutional neural networks (CNNs), are effective for image-based classification. They can be used to process sensor imagery and automatically classify airborne targets. AI also facilitates the integration and fusion of data from multiple sensors, including radar, infrared and visual sensors. This multi-sensor fusion improves the overall accuracy of target classification by considering a broader range of information.
Classification of Threat Level
Artificial Intelligence (AI) can be employed to assess and classify the threat level of airborne targets based on various factors. The system can define the threat perception as low, high or very high. The system can even ignore threats that are not so potent and focus on potentially dangerous targets.
Supervised machine learning algorithms can be trained on labelled datasets which may include information about different threat levels. These algorithms learn to recognize patterns in sensor data associated with varying threat levels, enabling automated classification of threats posed by each target. AI can even analyse the behaviour of airborne targets to identify patterns that correlate with certain threat levels. Unusual or suspicious behaviour, such as erratic flight patterns or sudden changes in altitude and speed, may be indicative of a higher threat level. AI enables real-time analysis of sensor data, allowing for quick threat level classification as airborne targets are detected.
AI systems can assist in continuously updating the threat-level classifications based on evolving patterns and emerging threats. It can even consider contextual information, such as the geopolitical situation, known threat profiles and the location of the airspace to refine threat level assessments.
Short-listing of Potent Targets
Machine learning models can be trained on historical data to recognize patterns and behaviours associated with different types of threats. These models can then prioritize airborne targets based on the likelihood of posing a significant threat. AI enables a dynamic flexible targeting strategy to shortlist the most dangerous airborne threats in real-time that are to be monitored and mitigated. The target selection can be facilitated by maintaining human oversight (humans in the loop), if it is essential. The system can continuously update target selection based on evolving patterns and emerging threats in real-time.
Data Fusion to Ascertain Potential Threats
Data fusion is a major element used to ascertain the findings/ inferences. Data fusion techniques merge radar and ESM sensors’ data to improve target accuracy, reduce false positives and enhance overall situational awareness. Data fusion can also facilitate calibrating and aligning data from different sensors to ensure consistency for learning purposes during peacetime. The existence of potential threats is never confirmed without involving data fusion techniques.
Tracking Potential Aerial Threats
Multi-platform/ multi sensor inputs are considered for the analytics to track multiple targets located at various locations. Tracking is a continuous process and the future positions of the aerial threats are iterated using Kalman filter algorithms. This will ensure that no target is missed in case of loss of data/sensor input due to hostile environmental conditions. AI-powered object detection algorithms, such as those based on convolutional neural networks (CNNs), can identify, localize and track multi-targets by taking sensor data.
Prediction of Future Course of Airborne Targets
Prediction of future course and the projectile of the targets is one of the most critical function. This prediction data should be accurate and critical since it becomes input to the next stage of mitigation of threats. AI models may consider environmental factors, such as weather conditions and terrain, which can affect the movement of aerial targets that may improve the accuracy of predictions. Predictive models can provide estimates of uncertainty associated with their predictions. Understanding the level of confidence in a predicted trajectory is also important for decision-makers.
Recurrent Neural Networks (RNNs) or Long Short-Term Memory (LSTM) Networks can be used for sequence modelling in time-series data. These architectures are well-suited for capturing temporal dependencies in the movement patterns of aerial targets. Kalman filters and Bayesian methods are used for dynamic state estimation and prediction. These techniques update predictions based on new sensor measurements, providing a continuous refinement of the predicted trajectory. Accurate prediction would certainly facilitate a better counter engagement in subsequent stages.
Target Prioritisation and Autonomous Selection of Launchpad and Counter Missiles
We can also use AI to prioritize targets based on their perceived threat levels at this stage. Factors such as the type of threat, its trajectory, potential impact and collateral damages are considered in this prioritization process. Decision-making algorithms can consider various factors, including the trajectory, speed and threat level of incoming objects. These algorithms autonomously assess the situation and determine the appropriate response.
The selection of most suitable launch platform for engaging the penetrating aerial target can be decided with the support of AI. Even the selection of the most suitable missiles/ method to counter the incoming missile/aircraft can also be entrusted to the algorithms. Humans in the loop can delay the process when multiple targets and ballistic missiles are penetrating the missile shield.
Based on the identified threat and the assessed risk, AI algorithms can autonomously select the appropriate kinetic or non-kinetic countermeasure. This could include launching anti-missile missiles, deploying electronic warfare measures or even activating other defensive systems. Autonomous systems can select the most suitable launchpad for launching countermeasures. Factors such as proximity, readiness, speed and the type of countermeasure available are taken into account.
Autonomous Missile Launch
We all are aware of LAWS (Lethal Autonomous Weapon System) and the campaign against them. Fully autonomous counter missiles come under the category of LAWS and are a necessary evil for autonomous air defence systems. Humans cannot be kept in the loop since the responses need to be quick and spontaneous. Once launched, these missiles are independent in making decisions to engage the potential aerial threat. The real-time analysis on board weapon allows the system to for dynamic response rapidly against evolving threat scenarios. Implementing adaptive AI systems that can learn and adapt to changing threat behaviours over time to respond effectively to evolving threats.
Confirmation of Mitigation of Targets
It is equally important to confirm the mitigation of every potential threat after a kinetic or non-kinetic kill while using autonomous systems. If there is a miss, then the threat scenario needs to be reassessed and the system has to re-engage the potential threat. The process will continue till the system confirms the mitigation of the threat. This functionality is very vital while countering a penetrating nuclear-tipped/ ballistic missile.
Conclusion
The development and deployment of autonomous air defence systems involve careful consideration of ethical, legal and safety implications. Transparency, accountability and adherence to international laws and agreements are critical aspects of responsible use in military or security applications. Regular testing, validation and updating of AI algorithms are necessary to ensure the reliability and effectiveness of the autonomous defence system against emerging threats. While the system operates autonomously, maintaining human oversight is essential. Human operators may have the ability to intervene, provide additional context or make high-level decisions based on their expertise and ethical considerations. Robust cyber-security measures are crucial to ensure the system’s integrity and prevent unauthorized access or tampering. Since we have a mix of various systems procured from many sources, the development of an optimal and effective air defence algorithm is a big challenge for our nation.
Disclaimer: The views and opinions expressed by the author do not necessarily reflect the views of the Government of India and Defence Research and Studies
Title image courtesy: Air Power Asia